
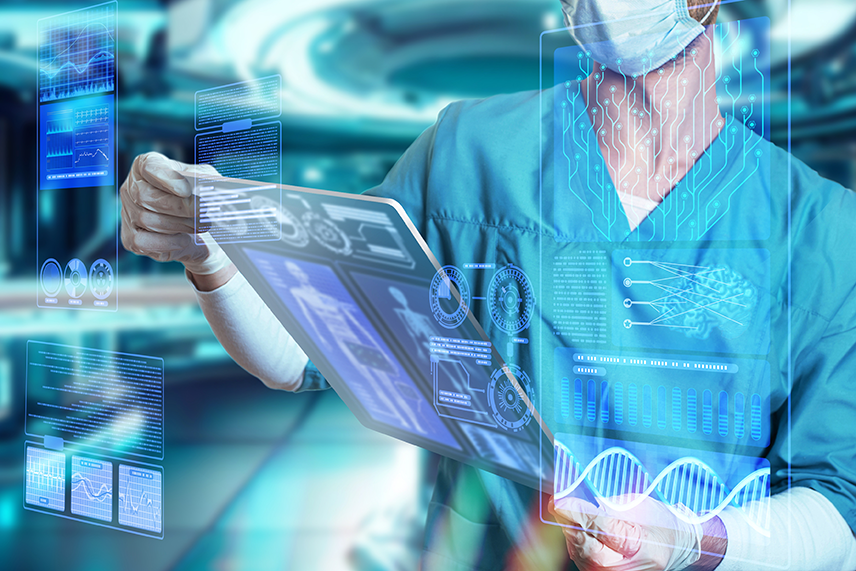
AI is accelerating innovation in healthcare and transforming the industry like never before. The applications of AI in healthcare are diverse, ranging from chatbot doctors to AI-assisted diagnosis and surgery to clinical analytics and healthcare management. Moreover, AI in healthcare benefits various groups: patients, physicians, providers, and payers.
The integration of AI in healthcare can help tackle some of the biggest burdens of the industry while improving patient care, enhancing operational efficiency, reducing cost, and advancing medical research. However, it requires careful consideration of regulatory and ethical concerns, data privacy, and ongoing monitoring and improvement of AI systems.
Most importantly, the successful integration of AI in healthcare demands a well-planned, multidisciplinary approach and effective collaboration among healthcare professionals, providers, and technology experts. Before we dive deep into the various use cases and applications of AI in healthcare, let’s learn what healthcare means.
What is Healthcare?
It is the organized provision of medical care to a community or individuals, with the aim of ensuring improvement in their health and well-being. This improvement is ensured by preventing, diagnosing, treating, ameliorating, or curing various ailments, diseases, illnesses, injuries, and other physical and mental impairments in people that affect the body or mind.
Healthcare services are rendered by health professionals and trained people in various settings such as hospitals, clinics, pharmacies, health centers, wellness hubs, camps, medicine stores as well as homes. However, they are shaped by social and economic forces such as availability, cost, quality, and fairness.
AI in Healthcare
With the enormous volume of multifarious parameters included in the definition of healthcare, machine learning, and AI naturally step in as new tools in the doctor’s toolbox. They help evaluate a vast volume of wide-ranging patient data and develop precise and personalized diagnosis and treatment plans.
This allows the generation of favorable patient outcomes while simultaneously ensuring the viability of the healthcare system. Thus, the entire healthcare system can be broadly viewed from these two different perspectives.
As per Statista, the global AI in healthcare market size is expected to reach almost $188 billion by 2030. Below is the range of the projected size for this market from 2021 to 2030:
17 Applications of Machine Learning and AI in Healthcare
Machine learning and AI have made significant inroads into healthcare. Here are some of the top applications of machine learning and AI in healthcare:
1. AI-Assisted Robotic Surgery
Machine learning and AI in healthcare have enabled the medical field to create and use collaborative robots in the process of surgery. The da Vinci Surgical System is a fine AI in healthcare example to look at. It is a robotic-assisted surgical device used by Mayo Clinic in the US for various treatments, including urologic, gynecologic, and colorectal surgeries.
The direction, depth, and speed of surgical robot movements can be precisely controlled. Surgical robots are well-suited for treatments that require repetitive movements. Robotic-assisted surgical devices enable healthcare professionals to carry out complex operations more accurately and precisely.
2. Clinical Diagnosis
Machine learning and AI in healthcare have significantly impacted the diagnostics vertical. Machine learning and AI algorithms can be trained to analyze CT scans, MRI images, X-rays, and other medical images to identify early signs of diseases like cancer. These algorithms can assist medical professionals in the early detection of a health problem, leading to faster diagnosis and effective treatment planning.
A Recent Meta-Analysis Showed That Machine Learning Algorithms Perform The Same Tasks As Human Experts, With 87.0% Sensitivity And 92.5% Specificity For Deep Learning Algorithms Versus 86.4% Sensitivity And 90.5% Specificity For Medical Professionals.
3. Medical Imaging Diagnosis
Newer medical devices for CT scans, MRIs, and X-rays make medical imaging better and help radiologists see where the health problem is. Researchers say pathologists look at and label many images of tissues to find out if a patient has a health problem. However, the chances of error could be higher in this case. This is where machine learning and AI in healthcare can help check the health problems shown by medical imaging.
For example, AI could find heart problems like a larger left chamber or complete tasks like measuring the aortic valve, the angle of the windpipe, and the width of the lung artery. UCSF Medical Center uses machine learning and AI for smart imaging. It has developed an AI system which uses deep learning methods to look at medical images and give suggestions to radiologists.
4. Electronic Health Record (EHR) Analysis
EHR is a digital snapshot of a patient’s medical journey, stored by doctors or healthcare providers. It contains all the relevant information about the patient’s care. Machine learning and AI in healthcare are like powerful magnifying glasses that help in managing and analyzing medical data to reveal hidden patterns and insights into the patient’s condition.
The Massachusetts General Hospital Clinical Data Science Center is a pioneer in using AI in healthcare for various purposes such as spotting diseases, diagnosing, treating, and managing them. The Center is using technology in different fields such as radiology and pathology and plans to expand their use of technology to EHR and genomics.
5. Improved Radiology
Radiology is a field where machine learning and AI in healthcare are very useful. Tumors, spots, and many other signs can show up in medical images at any time. It is easier to find and name these signs because the algorithms learn from many samples. The hard part is that these complicated formulas can’t show the location of the signs well, and this is where machine learning and AI can prove to be helpful. Many hospitals use AI-enabled radiology, like Mount Sinai Health System in NYC.
6. Drug Discovery
Drug development and production institutions also apply machine learning and AI to accelerate drug discovery, development, and production. By using the previously collected medical data on active ingredients in drugs and their combinations, machine learning algorithms can match each of them to fight diseases. For instance, XtalPi uses AI, quantum physics, and cloud computing on its ID4 platform to develop drugs.
7. Personalized Medicine
Medicine is a complex and resource-intensive specialty as every medical case is unique. Individuals usually have multiple conditions that need to be treated at the same time. Challenging and complementary decisions must be made to create an effective treatment plan that considers drug interactions and reduces side effects.
This is where machine learning and AI in healthcare becomes essentially important. For instance, the Cleveland Clinic in Ohio uses AI to customize healthcare plans with IBM Watson. It collects trillions of datapoints on patient records and simplifies precision medicine experience.
8. Precision Medicine
Precision medicine refers to medical treatment plans that have been customized for certain people or groups after genetic or molecular profiling to maximize effectiveness and therapeutic benefit. AI in healthcare can play a key part in precision medicine by the way of analyzing large amounts of complex data to identify patterns and associations that can inform personalized treatment decisions.
By doing so, it can help with clinical decision support by identifying treatment plans likely to give more effective health outcomes for individual patients.
An application that helps medical professionals plan radiotherapy and surgery, Microsoft’s Project InnerEye uses machine learning and computer vision to distinguish between malignancies and healthy anatomy using 3D radiological images. With this AI-based method, Microsoft aims to produce medicine specifically tailored to each patient’s unique needs.
9. Genome Sequencing
Genomics studies how genomes (the complete set of genetic material in a cell or organism) work, change, and map. Machine learning and AI in healthcare have helped genomics and genome sequencing a lot. They make the scientific discoveries in this field faster and help in:
- Putting together genomes.
- Finding differences between genome types.
- Developing new drugs and treatments based on genomic data.
A hospital in Toronto, Canada uses a machine learning-based method to find rare genetic diseases. AI-based ‘MendelScan’ uses machine learning to look at genetic data and search for possible mutations that cause diseases. It can also guess how likely a mutation is to cause a health problem in patients by comparing their genomic data to a database of known mutations causing diseases.
10. Clinical Research
Clinical trials and research are expensive and lengthy operations. It is logical for new drugs and medical methods to be tested for safety before being widely used, otherwise resulting in a loss of billions of dollars. There are situations, however, in which the solution must be made public as soon as possible.
In situations like the COVID-19 outbreak in 2019 or the Spanish Flu in 1918, information about a possible cure becomes the need of the hour. Machine learning algorithms have proved to be significantly important for collecting information on a large scale.
11. Disease Prevention, Outbreak, and Monitoring
Disease prevention and monitoring and predicting potential outbreaks are another area where machine learning and AI in healthcare have made an impact. By analyzing patient data, algorithms can detect disease risk factors and help doctors intervene before symptoms appear.
For example, machine learning can estimate which patients are at risk of developing diabetes and provide personalized suggestions for diet and exercise to prevent the disease. AI can also monitor the health of patients remotely, alerting doctors about the changes in the patients’ conditions before they become serious.
Moreover, the most recent COVID-19 outbreak has made everyone realize how unprepared the healthcare industry is if a disease of such magnitude breaks out. But thanks to machine learning and AI in healthcare, these technologies can help identify the early signs of an epidemic, ultimately preventing a pandemic.
12. Oncology Research
Cancer and death are strongly linked around the world. Even though things have gotten better in the last few years, cancer treatments and care still need to improve. Machine learning and AI in healthcare have helped give cancer patients more hope to prevent and manage cancer.
AI can help in oncology in many ways, such as:
- Improving clinical practice
- Supporting cancer research
- Understanding tumor features better
- Improving cancer patient results
- Improving treatment predictions
Furthermore, NLP can help find important articles and research papers, saving time and effort to look for treatment options. Pfizer, a premier biopharmaceutical company, has worked with IBM Watson to improve its immuno-oncology research. The two companies work together to accelerate the drug discovery process, study how the immune system fights cancer, and improve the results for cancer patients.
13. Virtual Nursing Assistants
Virtual nursing assistants are AI-powered assistants that can help healthcare professionals save time and focus on the key vulnerabilities of patients. A strong driver of the adoption of virtual nursing assistants is the shortage of medical labor which often creates pressure on healthcare workers who are available for service.
An AI-enabled virtual assistant can improve communication between the patient and the care provider, leading to better patient experience and reduced physical burnout.
14. Clinical Trial Optimization
Machine learning and AI in healthcare can do many things in research and clinical trials. Clinical studies take time, money, effort, and work. Researchers can use machine learning-based methods to identify, recruit, retain, and manage clinical trial participants in a fair and more efficient way.
Machine learning can also help get the right data and use electronic records to avoid data errors in clinical trials. Medidata, a unified life science platform, uses machine learning and AI to put data and AI-based thoughts together to make clinical trials and therapies better for patients. Its Rave EDC (Electronic Data Capture) can be used to capture and manage clinical trial site data, lab data, and patient data.
15. Behavioral Modification
Many companies have started to use machine learning and AI in healthcare in different areas like patient care, cancer prevention and detection, and behavior change. Behavior change is a therapy that tries to stop or reduce bad psychological behavior in children and adults.
Machine learning methods can look at past behaviors and suggest changes. For example, it can guess the chance of a person smoking, based on their data.
16. Predictive Analysis
Machine learning and AI can use medical data to make predictions in healthcare. Machine learning and AI-based methods can look at scores of data to find patterns and trends, and then use these ideas to guess what will happen next. Additionally, this area deals with hard data, but thanks to machine learning and AI, these technologies can expedite the process.
Kaiser Permanente, an integrated managed care consortium, uses predictions to find people who may have chronic diseases like diabetes or heart disease. Kaiser Permanente HealthConnect, the company’s tool for predictions, uses patient data to find risk factors by looking at a lot of data. It then gives personal help to patients to take care of their health.
17. Remote Patient Monitoring
With the availability of devices such as wearable sensors or smart watches, machine learning and AI in healthcare can prove to be extremely effective in monitoring patients remotely. They can help detect early warning signs of potential health issues, allowing for timely interventions.
8 Use Cases of AI in Healthcare Revenue Cycle Management (RCM)
It is a rule of nature that one must first survive if they are to save others. But who will save the savior – healthcare? Well, machine learning and AI play an equally important role as a savior when the question of viability or sustainability of the healthcare system arises.
By using AI and advanced analytics, healthcare organizations can not only ensure effective revenue management and visibility, but also, more importantly, healthcare for patients. Here are some examples of how AI can help modernize and improve RCM in healthcare by integrating the following capabilities into the workflow:
1. Predictive Modeling
Predictive modeling is the process of using data mining and machine learning algorithms to analyze data and make predictions about future events. In healthcare RCM, predictive modeling can enable organizations to find areas where they can improve their coding for better reimbursement.
For example, predictive modeling can help identify patients who are at risk of developing chronic conditions, thereby enabling healthcare providers to develop care plans to prevent or manage those conditions.
2. AI-Powered Real-Time Analytics
Real-time analytics using machine learning and AI in healthcare RCM can convert data into real-time insights. It can help healthcare organizations identify trends and patterns in their data as they come by. This enables healthcare organizations to make more informed decisions about their RCM processes and identify areas of improvement.
With trustable real-time data, healthcare organizations can speed up the decision-making process in their RCM. By leveraging data-based recommendations for performance and setting alerts when thresholds are not met, they can drive healthcare revenue cycle analytics best practice operations.
3. Automated Patient Payment Estimation
Patient payment estimation is the process of estimating how much a patient will owe for the healthcare services they receive. AI-powered automated patient payment estimation, along with automated eligibility and benefits verification, can help healthcare organizations improve their RCM by:
- Reducing the time and resources required to estimate and collect patient payments.
- Reducing the time and resources required to verify patient eligibility and benefits.
- Reducing errors and improving accuracy in the patient payment estimation process.
- Providing more accurate estimates of their out-of-pocket costs.
4. Automated Charge Description Management
Charge capture is the process of capturing charges associated with healthcare services. Charge description management is the process of managing the descriptions and codes associated with healthcare services. Charge capture and description management can be automated using AI, NLP, and rule-based technology.
With automated charge description management, healthcare organizations can improve their RCM by ensuring the descriptions and codes are accurate and up to date. They can also improve their RCM with automated charge capture by ensuring that all charges associated with healthcare services are captured and billed to the appropriate payers.
5. Automated Claims Verification
Automated claims verification is a function to automatically verify and process healthcare claims. With automated claims verification using AI, healthcare organizations improve their RCM by reducing the time and resources required to process claims. Furthermore, it can help reduce errors and improve accuracy in the claims process.
AI-based automated claims verification can be creatively designed to improve revenue collection by taking into account the eligibility of patients and verification of pre-authorization before the exam. With comprehensive claims reporting and web-based performance management tools, this helps create a flow of unmatched transparency.
Claim auditing is another area where AI can help. AI-based claim auditing helps ensure claims are accurate and comply with regulations. This, in turn, allows healthcare organizations to improve their RCM by reducing the number of denied claims and increasing the amount of revenue collected.
6. Automated Claim Denials Management
Automating claim denial management to transform RCM is one of the best advantages of implementing AI in healthcare as it helps:
- Maximize revenue.
- Reduce claim denials.
- Reevaluate claims management strategies.
- Ensure claims are paid without delay.
- Ensure claims are paid with higher accuracy.
AI-based automated claim denials management helps spot claims that have high chances of denial. This allows healthcare providers to intervene before the claims are submitted to reduce the risk of denial. Even when claims are denied, it can help find and segment denied claims that have the highest chances of approval once resubmitted.
7. Automated Physician Credentialing
AI in healthcare credentialing is a great way to automate, expedite, and streamline the process of verifying and validating provider credentials. It reduces the cost, time, and effort required for manual verification with real-time, automated verification of education, certification, and licensing details.
Furthermore, predictive analytics and AI can help detect fraud, gaps, anomalies, or discrepancies in provider credentials. Healthcare organizations can ensure the identity and integrity of provider credentials by using blockchain technology and biometric authentication.
This benefits healthcare organizations with improved RCM by ensuring only qualified physicians provide the services they offer to patients. Furthermore, based on best practices and evidence, AI can suggest optimal credentialing policies and procedures for healthcare organizations.
8. AI-Assisted Patient Outreach and Engagement
AI-enabled automated patient outreach can positively impact patient engagement and satisfaction, which goes a long way toward improving RCM. AI in healthcare helps:
- Sift through vast patient and health data.
- Pinpoint high-risk patients.
- Identify key risk factors for patients.
- Predict healthcare needs.
- Push out targeted, automated messages via multiple channels.
Moreover, AI can drive personalized patient experience. AI chatbots can automate patient communication, answer patient queries, recommend care, book appointments, and do more to enhance patient experience, which eventually promotes better RCM. Not to forget, AI and NLP can help improve health literacy as well as combat infodemic during pandemics like COVID-19.
Success Story: AI to Predict Readmission of Patients with Acute Coronary Syndrome
About the Client
The client is a healthcare software provider operating in the domain of cardiovascular and pulmonary diseases. They offer a customer relationship management solution to store and process patient data. Having significant acute coronary syndrome (ACS) data, they were looking to develop an AI-based healthcare solution to provide better services to not only hospitals and insurance providers but also patients.
Business Situation
Readmission is a critical measure of the quality of a healthcare system. It indicates poor care coordination or inadequate preparation for the post-discharge situation. For healthcare organizations, it has always been a top priority to lower readmission rates.
Thanks to AI-based intelligence solutions, they can help reduce readmission rates and avoid the associated financial penalties. The client was, therefore, looking for an efficient AI solution to predict readmissions in ACS patients.
Solution Overview
Harbinger developed an AI-based hospital readmission prediction system for the client by evaluating their ACS data and leveraging state-of-the-art machine learning algorithms. To build this AI solution, we used the following technologies and techniques:
- Data and analytics
- Readmission importance features graph
- Python-based model training
- XGBoost algorithm-based machine learning
- Flask API
- Gunicorn
- Docker
- AWS EC2 instance
Download to know the impact of Harbinger’s AI-based solution or learn more about this success story.
Parting Thoughts
It is time healthcare organizations and HealthTech companies start reimagining and redesigning healthcare and the technology it needs to provide better care to patients and improve the healthcare system. One way to achieve this goal is by leveraging AI and advanced analytics.
However, organizations need a partner who has done this successfully and can guide them with a customized solution approach for business use cases as highlighted in this blog post. This is where Harbinger comes in!
If you would like to explore forward-looking, future-ready, and intelligent healthcare management technology or HealthTech solutions, reach out to us at contact@harbingergroup.com. Schedule a free consultation with our HealthTech expert.